Matthew J. Daigle
About
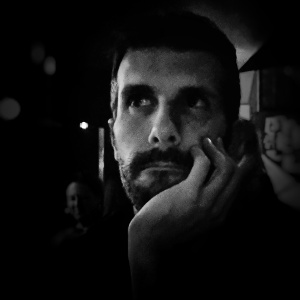
I am a research scientist working in the areas of artificial intelligence, machine learning,
and data science. While I have worked in diverse application domains and topics such as electric cars and aircraft, robotics, cryogenic propellant systems, simulation, and airspace safety, my main research emphasis is in systems health management, that is, I develop algorithms through which machines can self-diagnose their condition and predict their future failures.
Currently, I am a Principal Scientist at Novity, developing predictive maintenance software. Prior to joining Novity, I was a Principal Applied AI Research Scientist at PARC. Prior to that, I was a Principal AI Scientist at NIO USA, Inc., and a Research Computer Scientist and Lead of the Diagnostics & Prognostics Group at NASA Ames Research Center. Prior to that, I was an Associate
Scientist with the University of California, Santa Cruz at NASA Ames.
I received the B.S. degree in Computer Science and Computer and Systems Engineering from Rensselaer Polytechnic Institute, Troy, NY, in 2004, and the M.S. and Ph.D. degrees in Computer Science from Vanderbilt University, Nashville, TN, in 2006 and 2008, respectively. From September 2004 to May 2008, I was a Graduate Research Assistant with the Institute for Software Integrated Systems and Department of Electrical Engineering and Computer Science, Vanderbilt University, Nashville, TN.
Fun Fact: I have an Erdös number of 6, and a Bacon number of 3, resulting in an Erdös-Bacon number of 9.
Email: me at matthewjdaigle.com
Recent Publications
- G. Sierra Paez,
M. Daigle,
and K. Goebel,
“A Comparative Study on Computation of Cumulative Distribution Function in Predicting Time of Failure of Engineering Systems,” Annual Conference of the Prognostics and Health Management Society 2019, Scottsdale, AZ, October 2019. [show abstract]
Abstract:
- P. Ribot,
E. Chanthery,
Q. Gaudel,
and M. Daigle,
“Hybrid Particle Petri Net Based Prognosis of a Planetary Rover,” IEEE Transactions on Aerospace and Electronic Systems, September 2019. [show abstract]
Abstract:
- A. Bregon
and M. Daigle,
“Fundamentals of Prognostics,” Fault Diagnosis of Dynamic Systems, pp. 409-432, June 2019. [show abstract]
Abstract:
- M. Daigle,
A. Bregon,
and I. Roychoudhury,
“Diagnosis of Hybrid Systems using Structural Model Decomposition,” Fault Diagnosis of Hybrid Dynamic and Complex Systems, pp. 179-207, March 2018. [show abstract]
Abstract:
- Q. Gaudel,
E. Chanthery,
P. Ribot,
and M. Daigle,
“Diagnosis of Hybrid Particle Petri Nets: Theory and Application on a Planetary Rover,” Fault Diagnosis of Hybrid Dynamic and Complex Systems, pp. 209-241, March 2018. [show abstract]
Abstract:
- C. Kulkarni,
M. Daigle,
G. Gorospe,
and K. Goebel,
“Experimental Validation of Model-based Prognostics for Pneumatic Valves,” International Journal of Prognostics and Health Management, vol. 8, no. 1, December 2017. [show abstract]
Abstract:
Abstract: Prognostics is a systems engineering discipline focused on predicting end-of-life of components and systems. As a relatively new and emerging technology, there are few fielded implementations of prognostics, due in part to practitioners perceiving a large hurdle in developing the models, algorithms, architecture, and integration pieces. Similarly, no open software frameworks for applying prognostics currently exist. This paper introduces the Generic Software Architecture for Prognostics (GSAP), an open-source, cross-platform, object-oriented software framework and support library for creating prognostics applications. GSAP was designed to make prognostics more accessible and enable faster adoption and implementation by industry, by reducing the effort and investment required to develop, test, and deploy prognostics. This paper describes the requirements, design, and testing of GSAP. Additionally, a detailed case study involving battery prognostics demonstrates its use.
- G. Gorospe,
M. Daigle,
S. Sankararaman,
C. Kulkarni,
and E. Ng,
“GPU Accelerated Prognostics,” Annual Conference of the Prognostics and Health Management Society 2016, St. Petersburg, FL, October 2017. [show abstract]
Abstract: Prognostic methods enable operators and maintainers to predict future performance for critical systems. However, these methods can be computationally expensive and should be performed each time new information about the system becomes available. In light of these computational requirements, we have investigated the application of graphics processing units (GPUs) as a computational platform for general and real-time prognostics. Recent advances in GPU technology have reduced cost and increased the computational capability of these highly parallel processing units, making them more attractive for the deployment of prognostic software. We present a survey of model-based prognostic algorithms with considerations for leveraging the parallel architecture of the GPU and a case study of GPU-accelerated battery prognostics with computational performance results.
- M. Daigle,
I. Roychoudhury,
L. Spirkovska,
K. Goebel,
S. Sankararaman,
J. Ossenfort,
and C. Kulkarni,
“Real-Time Prediction of Safety Margins in the National Airspace,” AIAA Aviation Conference, Denver, CO, June 2017. [show abstract]
Abstract: Underlying all operations in the National Airspace System (NAS) is the concept of safety. Safety, as defined by acceptable levels of risk, is to be maintained at all times. The real-time safety monitoring (RTSM) framework is under development to provide an automated system to quantify safety in the NAS, estimate the current level of safety, and predict the future evolution of safety and the occurrence of events that pose an increased risk to flights so that these occurrences can be managed strategically rather than mitigated reactively. This paper presents the mathematical framework, the models, and the monitoring and prediction algorithms used to achieve this. RTSM computes safety as expressed through a set of safety margins based on user-defined safety metrics, thresolds, and events. Sources of uncertainty are modeled and propagated through the predictions in order to compute the probabilistic evolution of safety and the probability of events that introduce increased risk to operations. A prototype implementation is discussed and results demonstrating feasibility are presented. The results highlight the kinds of predictions that can be computed and the fidelity that is currently achieved.
- L. Spirkovska,
I. Roychoudhury,
M. Daigle,
and K. Goebel,
“Real Time Safety Monitoring: Concept for Supporting Safe Flight Operations,” AIAA Aviation Conference, Denver, CO, June 2017. [show abstract]
Abstract: Processes, procedures, regulations, and technologies are continuously evolving to maintain or improve the safety of the National Airspace System (NAS). In this paper, we describe a Real Time Safety Monitoring (RTSM) system that benefits from these efforts to define a set of safety metrics that are automatically monitored in real-time. In addition to providing information about current potentially adverse conditions to a variety of users, from those who need a broad overview of a day's flight operations to those who need to decide on a control tactic to employ in the next five minutes, the RTSM system predicts conditions within a specified prediction horizon. Its intelligent interface alerts the user, presenting the information as appropriate considering the current context and circumstances. We illustrate the system concept with five conceptual use cases, describing which safety metrics may be of the most interest to five user groups and suggesting a multi-modal display format. We posit that having access to information about adverse conditions in time to make efficient preemptive decisions without sacrificing safety will improve the already high level of safety and aid in the expansion planned for the NAS under the Next Generation Air Transportation System (NextGen).
Presentations